Engineering, Computing and Cybernetics
At the ANU College of Engineering, Computing and Cybernetics, you will be studying at Australia’s leading university – with a community of innovative students, teachers and researchers who are finding solutions to the world’s greatest challenges.
About
Study
Learn from our world-class researchers, as Australia’s policymakers do. Our expertise and influence extends to our Canberra neighbours, and leaders in government and industry.
Study with us
#1 for Employability
Be job-ready.
ANU is ranked first in Australia
and 35th in the world for Graduate Employability according to
the Global University Employability Ranking 2022.
#1 for Staff-to-Student Ratio
Be taught by the best. ANU is ranked first in Australia for the lowerst Student-to-Staff ratio among Australia's Group of Eight universities Global University Employability Ranking 2024.
Future Focused
Be innovative. ANU offers unique, modern degrees designed to meet the challenges of the future—preparing you for success in today's changing world.
Latest News

A First Nations voice in Engineering the future
17 Apr 2024
“I have a philosophy on life everyone has their own special strength. Find what you’re really good at and invest in it.” Mikaela Jade, CEO of InDigital.
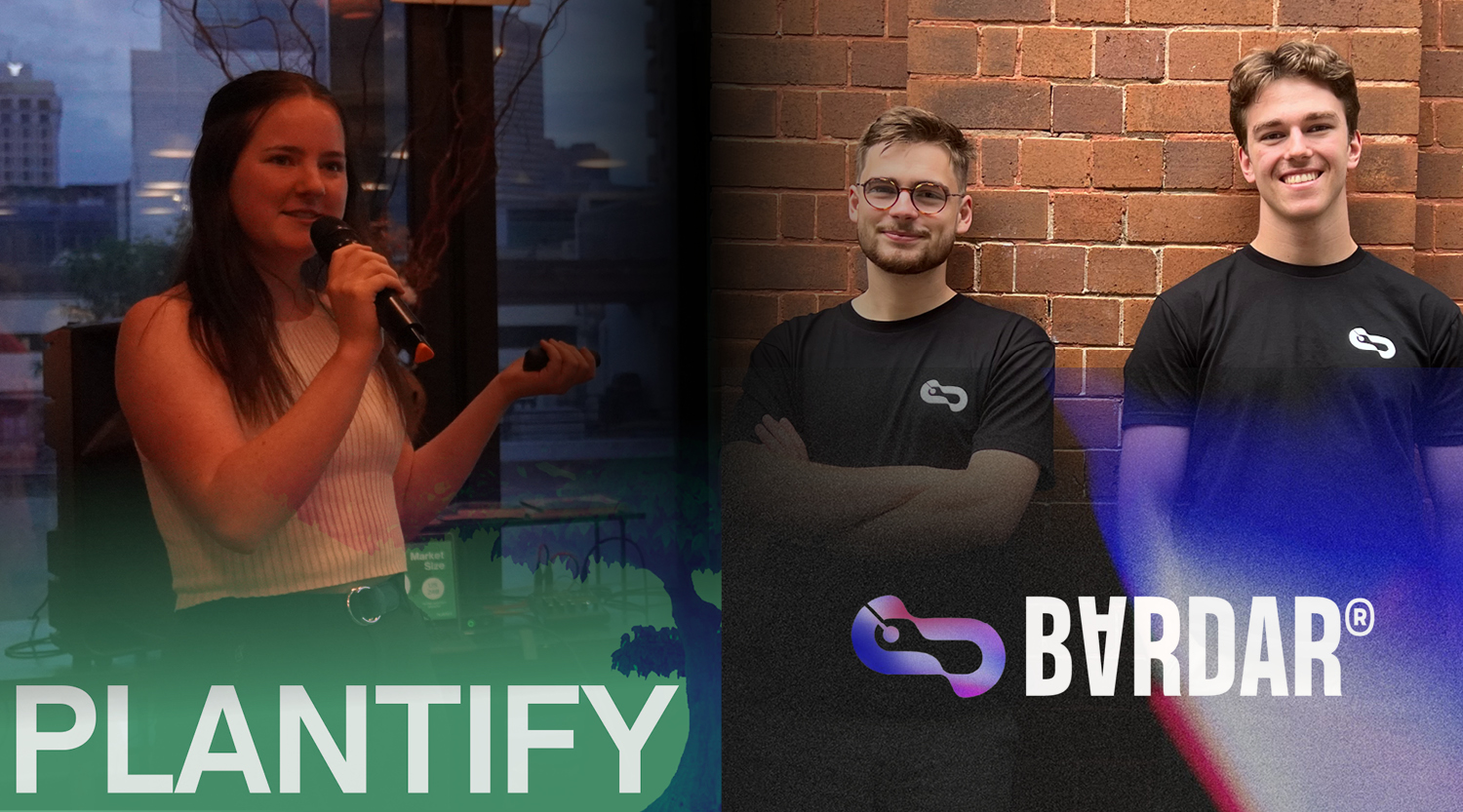
HEXperience of a lifetime – unveiling innovative student startups
10 Apr 2024
Earlier this year, a group of ANU students travelled to HEX International Singapore, a two-week immersive program for idea-stage founders to refine their start-up ideas and learn from leaders in business, social...
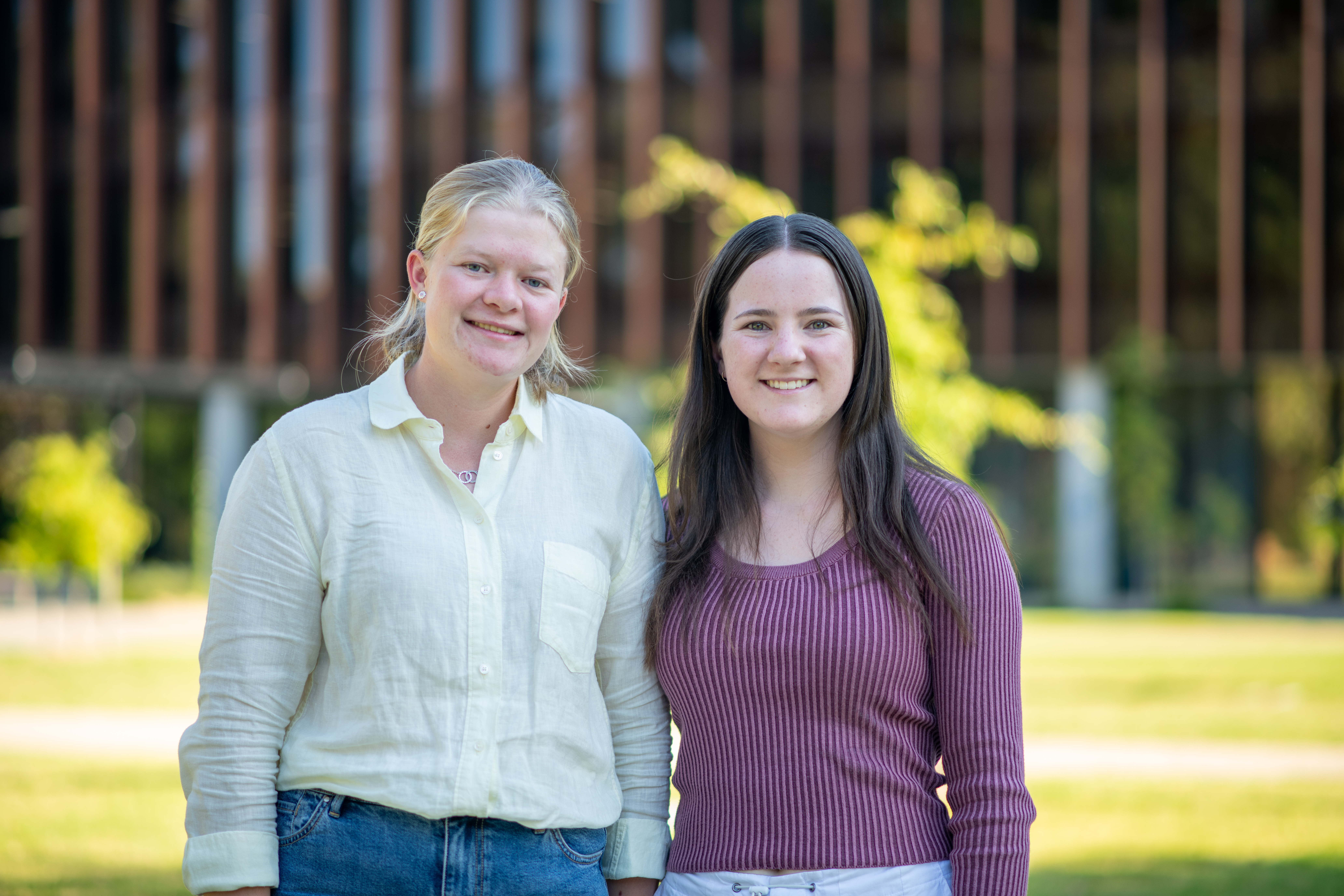
Natasha Linard scholarship recipients on engineering, leadership and women in STEM
26 Mar 2024
Growing up on a farm, Nikki Ballinger’s interest in engineering began at an early age - helping her dad out fixing machinery and learning how to weld and do woodwork. “I didn’t...
Invisible Section
Latest Events

Café Scientifique
23 Apr 2024
How do governments fit into the AI brew? Join us for a friendly chat on Artificial Intelligence and the role of governments!

Studying engineering & computing at ANU
2 May 2024
“There’s just one thing we ask of our students, that one day you will change the world. We will give you the tools, skills and the ability to go out and make...

ANU Computing Showcase Semester 1, 2024
28 May 2024
Join us and explore ANU Computing Work Integrated Learning Programs and Research Projects!